Data analysis is all about providing the evidence to answer the key evaluation questions you have about your program. In an objectives-based evaluation, this should clearly relate to your defined program objectives, so it is a good idea to review those again. Once you’ve decided what you are trying to say, and you have your data, your task is then to analyse this data to tell a story, which is most often about change.
In order to do that it is good practice to have some form of data dictionary or code book that will help you to understand the data you have obtained. This usually takes the form of a list of the questions asked with possible responses and coding. Negatively worded items may need to be reverse coded. A sample code book is provided below, demonstrating what this might look like for some demographic items and the first item of the Strengths and Difficulties Questionnaire (SDQ).
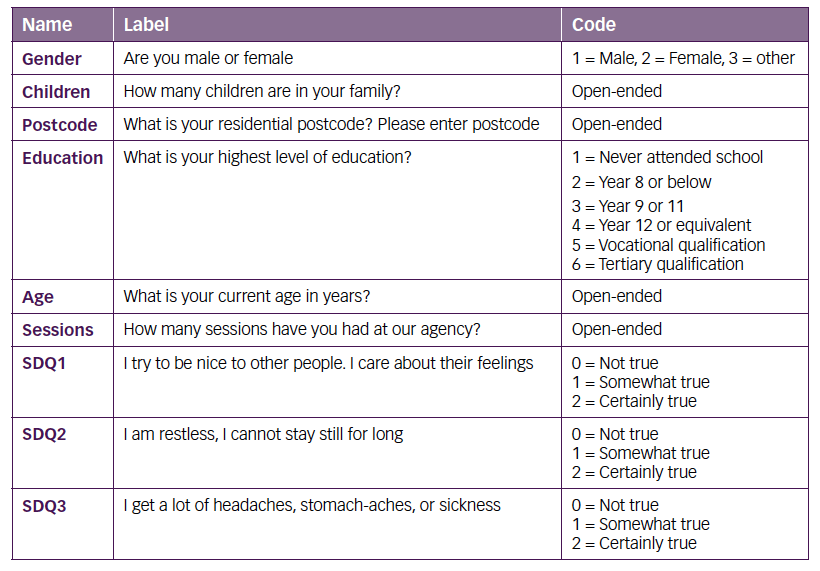
Once you have this, you can work with your data. Note that some measures require you to create subscale scores, which are usually the sum of responses to certain questions. This can be done relatively simply in a program like Excel, using the ‘SUM’ functions. For example, the PEEM has two subscales, including Efficacy to parent (11 items) and Efficacy to connect (9 items), as well as an overall measure consisting of all 20 questions.
Initial data analysis usually describing your results, using the measures of central tendency and spread discussed earlier. This will tell you about where your participants sit on various measures. It is good to be able to report these both before and after the program, so that you can demonstrate any changes.
For example: Prior to the program, participants reported feeling generally less confident and effective in their parenting. Scores are provided in the table below for before and after the program.
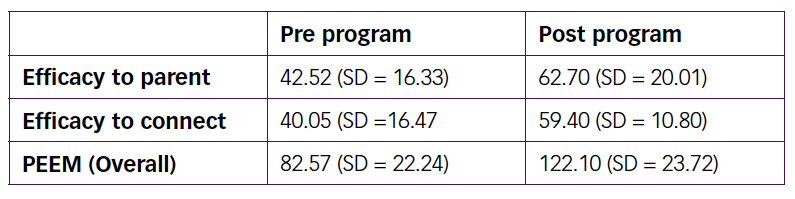
It appears from this information that the average scores have all increased in the desired direction, and that would suggest a strong program. However, in order to confirm this, a test of significance would be required. A test of significance (the p-value) can outline the probability that there was an effect produced by the program. A test of the effect size can then tell you the magnitude of the difference that has been produced. Whilst significance testing is complex, some simple tests can be done easily using Excel. Online tutorials are available to help you use these tests in Excel, and to advise you on what sort of tests to use. For the example above, a t-test would be used to determine whether the difference between the results is real, or just due to random chance. In this case, the difference is real and significant, and would be reported in the following way.
Participants reported an increased sense of efficacy in the parenting and connection, with an average difference of 39.5 (t=-5.3, p<.05).
Other relevant calculations include measures of correlation, which determines whether a shift in one value is associated with a shift in another value of interest, whether positive of negative. Using the sample above, it is logical to assume that age and number of children would be positively correlated, and this is usually reported as a Pearson’s correlation.
As expected, the number of children was positively correlated with age, with older women typically having more children (r=.65).
Other more complex analysis techniques help to compare more groups of data, or more than two sample points, and can also help to predict likely future values based on previous information. If you need this sort of analysis, we would recommend either employing a researcher skilled in these techniques, or accessing these services from an external provider or a research partner.